speak:wizARd
A hypothetical Augmented Reality based application for foreign language learners to practice their speaking skills.
Duration
Seven months
Role
UX Research, Ideation, Prototyping, User Testing, Statistical Analysis (SPSS)
Tools
Adoe Aero, Figma, Miro
Project Type
Research Project (Solo)
Project Brief: The goal of this project was to understand and compare the effects of feedback methods of AR in a foreign language speaking practice application that focuses on improving learners confidence for evaluating usability and workload.
Project Overview: I developed 2 AR prototypes with text based and expression based feedback in virtual practice scenarios to examine their effects on confidence of language learners and their foreign language speaking skills.
Project Outcome: I tested the 2 prototypes with 14 participants and derived insights by statistical analysis and qualitative analysis. Findings show learners inclination towards expression based feedback system.
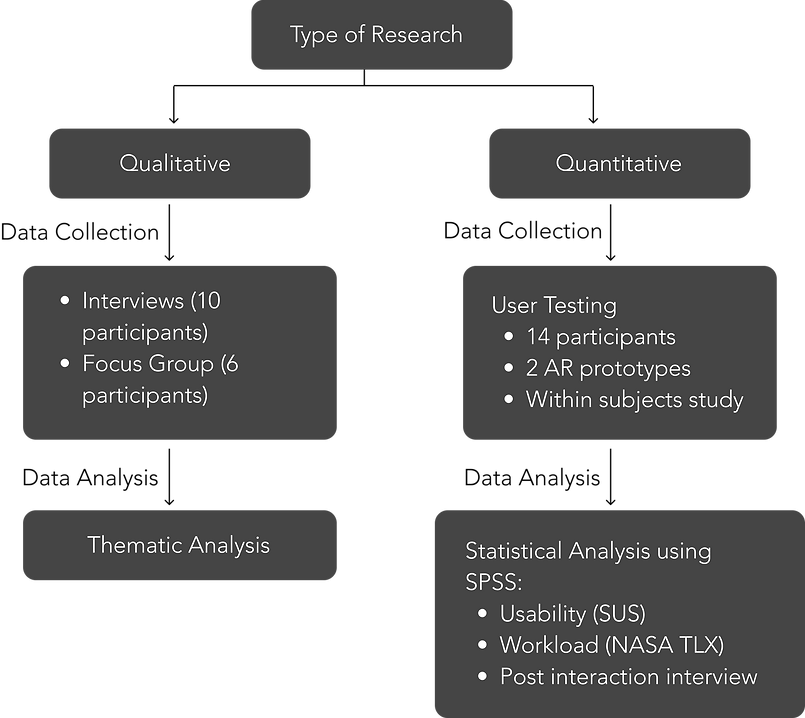
Initial research: Recognising the problem space
What problem am I solving?
Drawbacks of existing language learning resources like apps, websites and games:
Apps fail to keep learners motivated
Apps fail to provide a contextual environment
There is a lack of immediate feedback
Structured learning makes applications uninteresting
Existing research on foreign language learning using advanced technology:

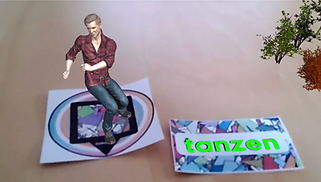
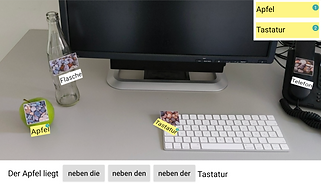
Wordsaber
​
A virtual reality (VR) edu- game for vocabulary learning.
cARdLearner
​
Demonstrates the possibility of using flashcard along with an expressive holographic agent on vocabulary learning.
Augmented Reality contextual learning
​
A handheld-AR app for learning case gram- mar by dynamically creating quizzes, based on real-life objects in the learner’s surroundings.
What is missing from the current research?
The research lacks focus on
- Praticing speaking skills
- Building confidence in speaking
- Guidance on how to make technological services more productive in learning.
I chose to focus on AR because:
- Easily accessible compared to VR. Can be used by anyone using their mobile.
- AR can bring the virtual side of VR into the real world. It helps create a better sense of reality over transporting the user into a completely foreign virtual world.
- Marker-based AR will support making the solution more contextual.
User Research: Empathise
Talking to the users
Interviews:
Sample: 10 participants
- Participants were learning and teaching different languages [French, Spanish, Hindi, English and German].
- Participants had different learning backgrounds (native language), tools and motivations.
Goal
3 Foreign language tutors: To understand their teaching strategies and readiness to adapt advanced tools into the teaching process.
​
7 Language learners: To explore various experiences of learners and understand the impacts of different learning methods on confidence and speaking skills + guaze their willingness to incorporate AR/VR solutions in the learning process.
Focus Group:
Sample: 6 participants
Activities conducted:
1. Discussing the motivations and challenges of learning a foreign language.
2. Breakdown of the Duolingo app
3. Discussing users experience with game based learning.
Goal
To answer the question: Can game-based learning impact progress and motivation of users to learn a Foreign Language?


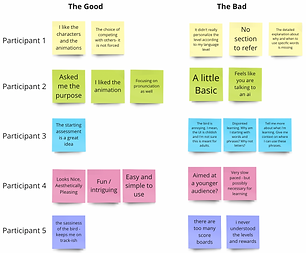
What do the users say?
Thematic Analysis of the interview data was conducted manually to analyze the interview data.
​
Steps: 1. Familiarizing yourself with the data
2. Generating codes
3. Searching for themes
4. Reviewing potential themes
5. Defining and naming themes
6. Reporting the themes
Sub-theme
Theme
Access to people to practice speaking
1. Fear of being judged: A personality trait
Personality dictates practice approaches
"if somebody talk to me in some English questions I don't understand and, and they just look straight at me. I think we all feel very nervous."
Having no other choice is a positive
2. Simple accomplishments boost confidence
"In Germany […] search for the like the city centre […] my initial action was to ask him in English, but then he did not understand. […] I asked him in German and then he answered. I felt so good."
Lack of context
3. The app and the learner are not on the same page
"some native speakers speak words different to the way they teach us. So I think maybe the most difficult is to get used in the real life.”
Misleading generic translations
Correlating foreign language to their native tongue
4. Importance of familiarisation with cultural nuaunces
, "So German originated from Sanskrit and, like Marathi, […] So that really helped me remember […] understand the actual meaning […] some words explain emotions or there is a particular essence for that […] easy to understand by comparing […]."
What did I take away from this research?
Insights:
1. Game-based learning sounds interesting, but it does not guarantee learning.
2. People's preferred learning/ practice methods/ approaches are based on their confidence and personality. This affects their engagement with the social features of game-based learning.
"It gives me words and rewards for remembering them but what am I supposed to do by practicing saying "that is a boy" again and again?" laughs.
"When it is about speaking a language I don't need a leader-board to tell me where i am compared to other users. The focus needs to be on me."
Ideation: Wireframing
How am I proceeding with the insights?
Sketching initial wireframes from user ideas.

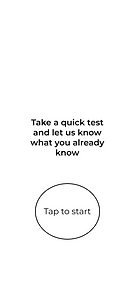
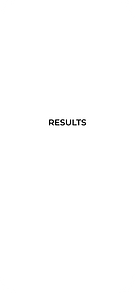






Feedback from initial user testing:
1. Modify the language of the application. For example, change "take a quick test to let us know what you already know" to "lets take a quick quiz to test your skills".
​
2. Show examples of what the test would look like and what kind of results should users expect on the screen.
​
3. Add an instruction screen before entering the AR view specifying beforehand what tasks needs to be performed in the AR scenario.
​
4. Show what progress will be saved.
Feedback 1 and 2 modified user flow:
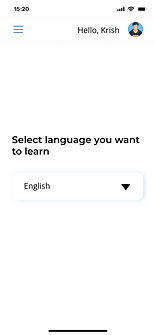
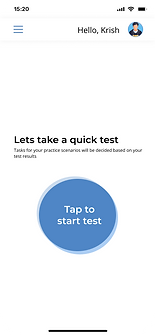
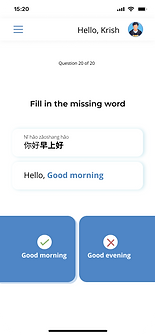
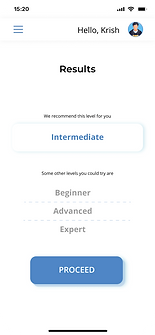
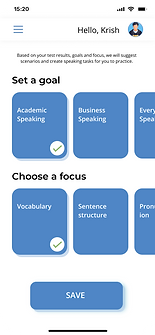
Feedback 3 and 4 modified user flow:
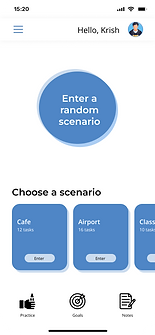
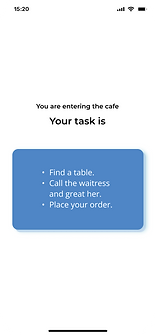
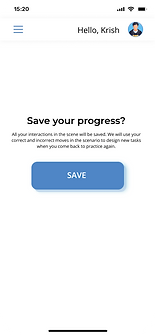
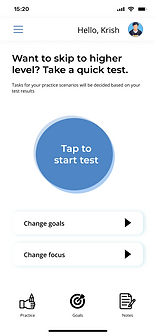

Ideation: defining the features
How am I addressing user needs?
I developed features for the app by considering various behavioural and game design theories
Solution
Need
Success opportunity
Control
Social isolation
Active engagement and opportunities to increase mastery
1 Encouraging feedback about improvement
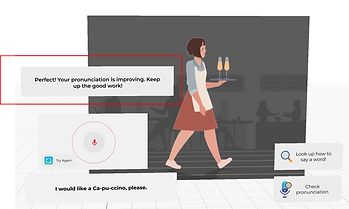
2 Corrective (Direct) feedback for self practice
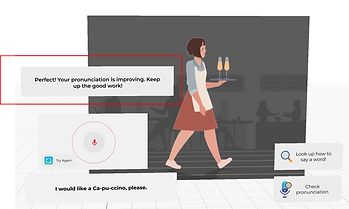
3 Corrective (In-Direct) feedback for self practice
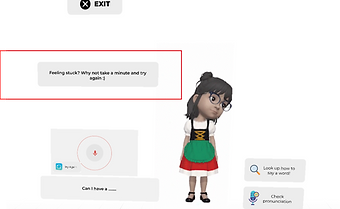
4 Immersive real time response with feedback

5 Scenario generation based on feedback
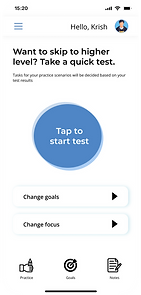

User Testing: developing the AR world
How did I test the AR prototype?
In case of scenario-based learning, the level of immersion is determined by how the characters communicate. I wanted to test two different AR feedbacks.
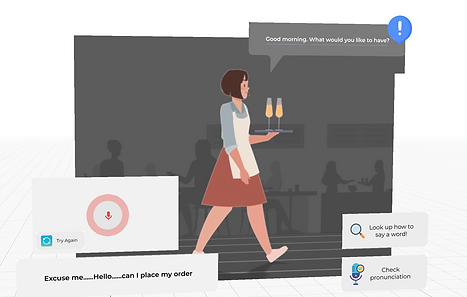
Prototype A: Stationary character in the virtual environment.
Feedback in the form of text in the virtual view.
Sample: 14 people (due to time restrictions)
All the participants were langusge learners with an experience of using apps and websites to study the foreign language of their choice.

Prototype B: Interactive animated characters.
Feedback in the form of facial expressions matching the text.

Method: Within Subjects study
Number of groups: 2
Number of prototypes; 2
Factors to be tested: SUS, NASA TLX
1. Breifing the participants about the process and scales to be used.
2. Participant interacts with first prototype and completes the task.
3. Fill the SUS scale for usabilty and NASA TLX scale for workload for the first prototype.
4. Participant interacts with the second prototype and completes the task.
5. Fill the SUS scale for usabilty and NASA TLX scale for workload for the second prototype.
6. A short post interaction interview to understand the impact of both prototype on confidence.
User Testing: Analysing the data
How did I analyse the collected data?
Quantitative statistical tests : Using SPSS
Usability
Workload
Mental Demand
Mean
prototype A = 73.75 (Std. Dev= 10.22) < prototype B = 76.07 (Std. Dev= 11.03)
​
Participants found prototype A more usable.
prototype A = 40.66 (Std. Dev= 15.65) > prototype B = 33.23 (Std. Dev= 16.73)
​
Participants found prototype B has less workload.
prototype A = 45 (Std. Dev= 20.93) > prototype B = 38.92 (Std. Dev= 24.19).
​
Participants found prototype B less demanding.
Test of normality
statistical significance is 0.511 > 0.05
​
Data is normally distributed
statistical significance is 0.289 > 0.05
​
Data is normally distributed
statistical significance is 0.212 > 0.05
​
Data is normally distributed
Paired sample T-test
p= 0.397 > 0.05
​
No significant difference in usability of both prototypes.
p= 0.062 > 0.05
​
No significant difference in workload of both prototypes.
p= 0.218 > 0.05
​
No significant difference in mental demand of both prototypes.
Quantitative statistical tests : Using SPSS
1
13/14 participants preferred the expression based interaction (Prototype B) saying the expressive avatar feels more friendly and gives a sense that it cares about the user
"I'm a visual learner […] seeing the interaction happen instead of just text, that is interesting. Making things a bit futuristic is always interesting […]." -Participant 2
2
Participants preferrence can change depending on the situation, like, time available, urgency, level of expertise etc.
"as a beginner I would not care about the expressions, I just want the answers. But when at a certain stage […] looking for interesting ways to learn the expressions will be helpful".
- Participant 5
3
Technical solutions cannot guarantee a change in your personality that determines your confidence in real life situations.
"I can see this app helping me learn better, but confidence is my own thing, so even if I learn, having to actually speak in real life will completely depend on me in that moment."
-Participant 1
What are the limitations of this research?
1
The limited number of participants for testing makes the statistical results less reliable. Time restrictions contributed to the decision to test with limited participants.
2
I was unable to recruit participants all learning the same language at the same level. This let to designing a generic prototype meaning I could not numerically measure any difference in the users speaking skills before and after interacting with the prototypes.
3
I decided to not consider the participants familiarity with using AR as this would have made eligibility more rigid. This contributed to the insignificance in workload and mental demand scores of both prototypes.
4
The similarity in the tasks to be performed during both interactions and the minimal design along with carryover effect of within subjects study also contributed to the insignificant different.
How can we improve the research in the future?
The research needs to take a more experimental approach with a measurable entity conducted over a longer period of time.
​
Further research should also recruit more participants learning the same language and developing an advanced prototype for testing one particular language.
​
Two approaches can be take:
1
Create tests for before interaction and after interaction so as to guage the effects of specific elements of the prototype by comparing the difference in test scores.
2
Divide participants in two groups where one group interacts with the AR prototyes whereas the other group studies in traditional classroom textbook setting. Then compare differences using tests.
Does this research raise additional questions?
This research and its findings presents some new research questions that can be considered for further development:
​
​
1. How can we implement negative and corrective feedback non-threateningly using expressions?
The objective will be to understand the effect of this feedback on learning progress and how facial expressions can be leveraged positively in the learning process. One way to do this is by developing highly rendered expressive characters and testing users' responses to different facial expressions.
2. How can location-based AR be developed to customize the gamification and scenario suggestions more customized to each user? Understanding this will help cater to specific regions' vocabulary, accents, and speaking styles. Researchers must gauge the AI's ability to create extensive and personalized scenarios to achieve this.